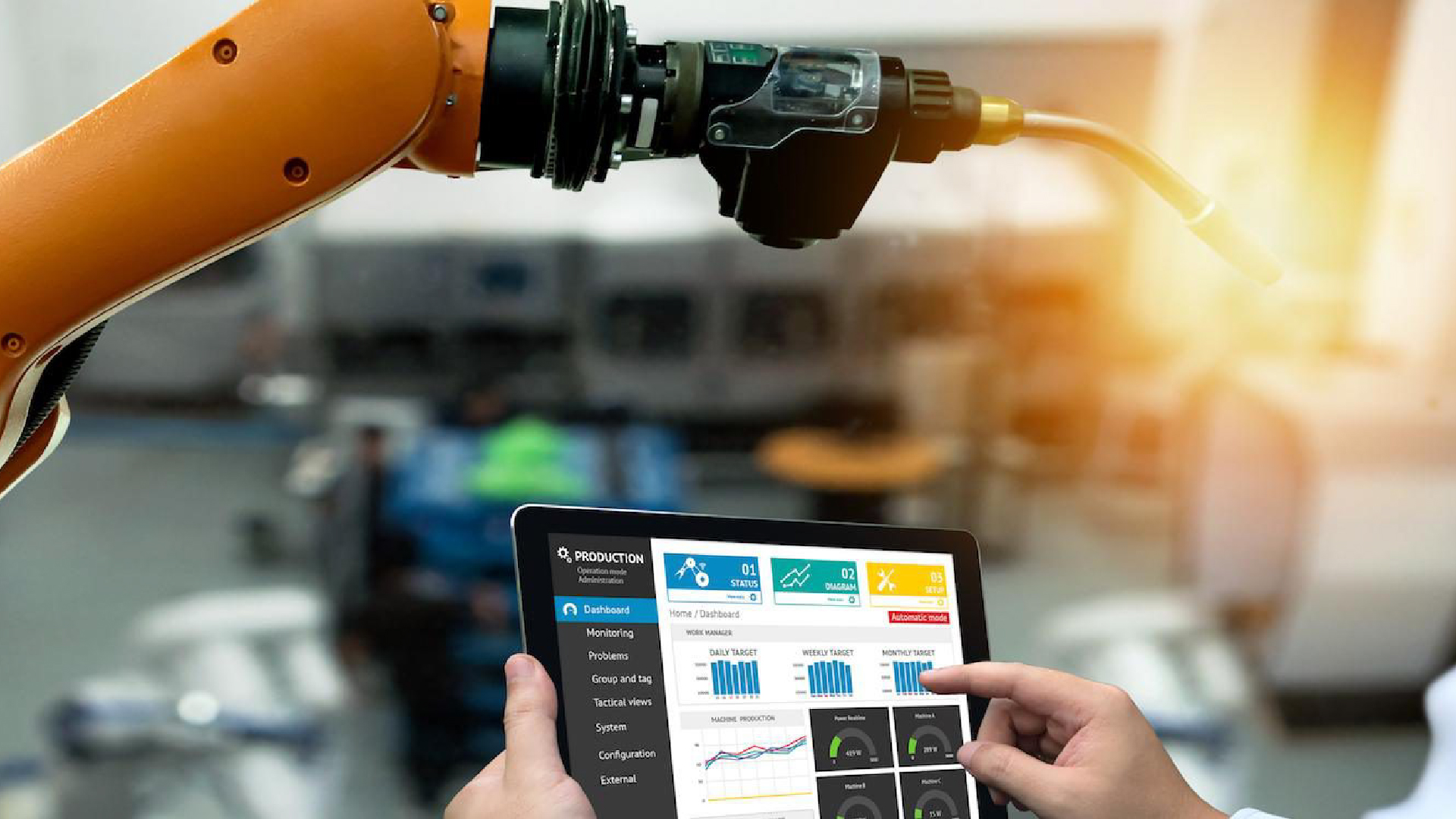
Intelligent Automation for Work and Life
At Quantilus, we have been working with AI before it became cool (and scary). Our first foray was in the field of Natural Language Processing – which we used for automated grammar and style checks of written content. Subsequently, we built tools to classify untagged content in intelligent, usable ways, and to present it for consumption with a high degree of personalization. More recently, we have been working on personality assessment of individuals based on 1) the words they speak (a relatively simple task), and 2) changes in their facial patterns based on verbal and visual cues (a much more complex task).
Want to build Virtual Reality or Augmented Reality apps for your business? We built some of the first business-focused AR apps for mobile and wearable platforms through our SAP partnership. Our apps help technical support personnel visualize product models, and also let customers visualize retail products in empty space. With the added complexity of tight integration with backend ERP systems.
FEATURED WORK
TECHNOLOGY STACK
Some of the frameworks and tools that our development teams have used recently. A list that grows by the day.
RELATED RESEARCH
Relevant, interesting and current curated research content in the field.
Explaining Artificial Intelligence to Your Teenager
Although it’s an older movie, a lot of teens have seen The Terminator and know what happens if you’re busy playing video games instead of preparing for SkyNet. That world’s AI isn’t too far from what’s available today—but what, exactly, is artificial intelligence? And how can you explain it to a younger generation?
Let’s start with its history. In 1956, Marvin Minsky and John McCarthy, who coined the term AI, described it as a task performed by a machine or program that—were a human to do the same task—would require at least some intelligence to complete. The definition has evolved somewhat in the past 68 years, but generally all AI systems include the following behaviors which we associate with human intelligence:
- Planning
- Learning
- Reasoning
- Knowledge representation
- Perception
- Problem solving
- Manipulation
- Social intelligence
- Creativity
Two types of artificial intelligence
There are two types of AI: narrow AI and general AI. It is the narrow AI that permeates our world today, in all fields from medical to mechanic, financial to engineering, and everything in between. General AI is still a ‘pipe dream’ that computer engineers are working to creating. Most experts say we’re still a decade or two away from achieving true general AI because of its complexity.
Narrow Artificial Intelligence
Most computers use narrow AI. They’re intelligent systems that know how to conduct specific tasks without having been explicitly programmed to do so. Apple’s Siri is a perfect example. So is Amazon’s Alexis, Google’s new virtual assistant, and IBM’s Watson supercomputer.
These systems simulate a human being’s knowledge and cognitive ability within specific parameters. The systems can include self-driving cars and spam filters. Why? Because the systems use pattern recognition, natural language processing, machine learning, and data recognition to make decisions.
Narrow AI, in addition to telling you a joke or the weather, has a host of applications. These systems can identify inappropriate content online or in emails, respond to customer service requests, read video feeds from drones, organize and coordinate business/personal calendars, analyze data to make predictions, and more.
General Artificial Intelligence
This AI—also referred to a human-level, strong, and superintelligence AI—can understand and reason within its environment, just like a human. Think Data from Star Trek: The Next Generation, or Hal from 2001 A Space Odyssey.
It’s “strong” because this AI will be stronger than us humans and “general” because we’ll be able to apply it to all problems. However, it’s nearly impossible to create a computer that can think abstractly, innovate, or plan. Experts agree that it’s really difficult—at this point still impossible—to teach a computer how to invent something that doesn’t exist.
AI is gaining strength—it can produce more accurate predictions about the data it’s fed. That DeepMind algorithms can win more games and transfer learning from one game to another is another indication that AI is growing stronger.
Whole Brain Architecture Approach
Dr. Hiroshi Yamakawa, Director of Dwango AI Laboratory, is one of the world’s foremost authorities on AI. He says that currently, AI can solve particular issues or address specific problems. His organization is using the Whole Brain Architecture Approach which is an engineering-based approach to “create a human-like artificial general intelligence (AGI) by learning from the architecture of the entire brain.” This AGI uses the human brain’s hard wiring as a model to integrate machine learning modules and artificial neural networks. He theorizes that the WBAI will be achieved by 2030 and will help to find solutions for global problems that include environmental, food, and space issues.
Still a journey to achieve artificial general intelligence
Computer scientists continue to work to develop an actual AI that can think like a human. We’ve seen the “results” of such successes in Terminator, I, Robot, A.I. Artificial Intelligence, Ex Machina, Blade Runner, and many other sci-fi books movies and books.
But the reality is that even the world’s best machine learning engineers, with access to millions of dollars, are struggling to build a general AI product. Nearly $15.2B of capital venture was given to AI startups in 2017 and over 45,000 research papers on AI have been published since 2012. Read this article to learn more about what’s propelling the recent surge in general AI development.
What’s next?
The closest thing we’ve got today to general AI is machine learning (ML). This term describes feeding vast amounts of data into a computer system which then extrapolates it to carry out a specific task—like Facebook’s algorithms that can recognize faces from your contacts list or Waze and Google Maps, that can analyze traffic speeds and plot alternate routes. And there are many other examples of machine learning, a growing field designed to create machines that are faster and more accurate.
How does machine learning work?
In a nutshell, this subset of AI uses statistical techniques to enable a computer to learn without explicit programming. According to Dr. Yoshua Bengio, from the University of Montreal, machine learning uses data, observations, and world interactions to provide computers with acquired knowledge which then facilitates the computers’ ability to accurately generalize to new settings.
ML groups a variety of algorithms by learning style or similarity in form or function. These algorithms include representation, evaluation, and optimization—their goal is to provide computers with the “skill” to interpret never-before-seen data and apply it to new situations.
The field of ML and data science continues to grow, but while these mathematical concepts can be implemented into real world applications, this so-called deep learning isn’t real intelligence… yet. It’s a type of mathematical optimization that does have limits. The “thinking” is limited to specific domains and the intelligence depends on the training dataset (so humans are still in control). It’s difficult to use it within constantly-evolving, dynamic environments and can’t be used for control problems—only classification and regression. And to ensure the greatest accuracy, it requires huge datasets.
Will we ever achieve true artificial intelligence?
It’s hard to say. Sixty-two years after its inception, we’re still working to achieve true AI. Weak AI systems make more and more decisions as scientists and engineers develop ways to gather, quantify, and feed more data into more algorithms.
And we must, caution Phil Torres, an Affiliate Scholar at the Institute for Ethics and Emerging Technologies, consider the human element—as AI develops, it’s incumbent upon those in the field to program human values into algorithms. After all, he says, “If we suddenly decided, as a society, that we had to solve the problem of morality—determine what’s right and what’s wrong, and feed it into a machine—in the next 20 years… would we even be able to do it?”
What is Machine Learning and How Will It Impact Business?
It’s a bit difficult to narrow down one specific definition of machine learning (ML), because you’ll get a different explanation depending on whom you ask.
Nvidia defines it as “the practice of using algorithms to parse data, learn from it, and then make a determination or prediction about something in the world.” McKinsey&Company agree with Nvidia, saying that ML is “based on algorithms that can learn from data without relying on rules-based programming.” Stanford suggests that ML is “the science of getting computers to act without being explicitly programmed.”
And Carnegie Mellon’s definition—also a favorite of many other experts in the field—states “the field of Machine Learning seeks to answer the question ‘How can we build computer systems that automatically improve with experience, and what are the fundamental laws that govern all learning processes?’”
Regardless of the definition you choose, at its most basic level, the goal of machine learning is to adapt to new data independently and make decisions and recommendations based on thousands of calculations and analyses. It’s done by infusing artificial intelligence machines or systems with the ability to learn from the data they’re fed. The systems learn, identify patterns, and make decisions with minimal intervention from humans. Ideally, machines increase accuracy and efficiency and remove (or greatly reduce) the possibility of human error.
The importance of machine learning
The nearly limitless quantity of available data, affordable data storage, and growth of less expensive and more powerful processing has propelled the growth of ML. Now many industries are developing more robust models capable of analyzing bigger and more complex data while delivering faster, more accurate results on vast scales. ML tools enable organizations to more quickly identify profitable opportunities and potential risks.
The practical applications of machine learning drive business results which can dramatically affect a company’s bottom line. New techniques in the field are evolving rapidly and expanded the application of ML to nearly limitless possibilities. Industries that depend on vast quantities of data—and need a system to analyze it efficiently and accurately, have embraced ML as the best way to build models, strategize, and plan.
Industries that use machine learning
Healthcare. The proliferation of wearable sensors and devices that monitor everything from pulse rates and steps walked to oxygen and sugar levels and even sleeping patterns has generated a significant volume of data that enable doctors to assess their patients’ health in real time. One new ML algorithm detects cancerous tumors on mammograms; another identifies skin cancer; a third can analyze retinal images to diagnose diabetic retinopathy.
Government. Systems that use machine learning enable government officials to use data to predict potential future scenarios and adapt to rapidly changing situations. ML can help to improve cybersecurity and cyber intelligence, support counterterrorism efforts, optimize operational preparedness, logistics management, and predictive maintenance, and reduce failure rates. This recent article highlights 10 more applications for machine learning within the healthcare industry.
Marketing and sales. Machine learning is even revolutionizing the marketing sector as many companies have successfully implemented artificial intelligence (AI) and ML to increase and enhance customer satisfaction by over 10%. In fact, according to Forbes, “57% of enterprise executives believe that the most important growth benefit of AI and ML will be improving customer experiences and support.
E-commerce and social media sites use ML to analyze your buying and search history—and make recommendations on other items to purchase, based on your past habits. Many experts theorize that the future of retail will be driven by AI and ML as systems become even more adept at capturing, analyzing, and using data to personalize individuals’ shopping experiences and develop customized, targeted marketing campaigns.
Transportation. Efficiency and accuracy are key to profitability within this sector; so is the ability to predict and mitigate potential problems. ML’s data analysis and modeling functions dovetail perfectly with businesses within the delivery, public transportation, and freight transport sectors. ML uses algorithms to find factors that positively and negatively impact a supply chain’s success, making machine learning a critical component within supply chain management.
Within logistics, ML facilitates the ability of schedulers to optimize carrier selection, rating, routing, and QC processes, which saves money and improves efficiency. Its ability to analyze thousands of data points simultaneously and apply algorithms more quickly than any human enables ML to solve problems that people haven’t yet identified.
Financial services. The insights provided by ML in this industry allow investors to identify new opportunities or know when to trade. Data mining pinpoints high-risk clients and informs cybersurveillance to find and mitigate signs of fraud. ML can help calibrate financial portfolios or assess risk for loan and insurance underwriting.
The future of AI and ML in this industry includes an ability to evaluate hedge funds and analyze stock market movement to make financial recommendations. It may render usernames, passwords, and security questions obsolete by taking anomaly -detection to the next level: facial or voice recognition, or other biometric data.
Oil and gas. ML and AI are already working to find new energy sources and analyze mineral deposits in the ground, predict refinery sensor failure, and streamline oil distribution to increase efficiency and shrink costs. ML is revolutionizing the industry with its case-based reasoning, reservoir modeling, and drill floor automation, too. And above all, machine learning is helping to make this dangerous industry safer.
Manufacturing. Machine learning is no stranger to the vast manufacturing industry, either. It’s accomplishing the goal of improving operations from conceptualization to final delivery, significantly reducing error rates, improving predictive maintenance, and increasing inventory turns.
Not unlike the transportation industry, ML has helped companies improve logistical solutions that include asset, supply chain, and inventory management. It also plays a key role in enhancing overall equipment effectiveness (OEE) by measuring the availability, performance, and quality of assembly equipment.
ML & AI: here to stay
Is all the hype surrounding machine learning really worth it? Most experts say “yes” – with this caveat: The key is understanding how to use it to meet each individual business’s challenges and goals. It’s clear, based on a significant volume of data and evidence, that machine learning and artificial intelligence are here to stay. The trick, however, is recognizing that ML and AI aren’t a magic spell that work for every situation.
Experts agree that it’s important to clearly understand the value that incorporating ML will bring to your business. If it’s negligible, the expense may not bring a significant enough return on investment (ROI). This article from Business highlights four questions to ask before you consider beginning an ML project.
The Evolution of Artificial Intelligence in Automated Interviews
An emerging trend in recruiting is the rise of automated interviewing, including the integration of artificial intelligence technology. This transition is a boon for the largely outdated recruiting industry. AI components allow companies to easily sift through dozens (or hundreds) of interviews quickly with objective metrics and consistent results. But as the rise in AI automation becomes more widespread, many readers may ask themselves, “how did artificial intelligence make its way into the hiring process in the first place? Isn’t hiring a fundamentally human process?”
Benefits of Artificial Intelligence in Recruiting
The emergence of new technologies in the recruiting space has been met with plenty of skepticism over the years. However, the advantages of this new technology far outweigh the fear of AI’s ability to understand and analyze human behavior. Utilizing AI in recruiting brings many benefits for organizations in every industry. These include:
- Saving time by automating tasks
- Standardizing assessment across the board
- Improving the candidate experience
- Decreasing turnover and hiring costs
(Watch out for a blog post from us soon detailing exactly what benefits your company will find with AI automated interviews.)
For a nonprofit organization, small business, or startup, the cost of a bad hire could be the make or break point for their company. With the average cost of a bad hire ranging from $25,000-$50,000 (and beyond), the stakes are high to recruit the best talent as quickly as possible.
Current State of the Industry
The hiring and recruitment process has remained largely stagnant for decades. In fact, a recent study by Fast Company showed that the processes from application to acceptance are becoming even less efficient, increasing from 13 days in 2011 to nearly a month today. This equates to lost productivity and a greater strain on teams who are missing staff while searching for quality talent.
In the past two years, artificial intelligence has become a trending topic in the recruiting space. AI has been utilized in the hiring process by applying techniques like natural language processing and facial expression recognition. Natural language processing analyzes the word choice and patterns an individual uses to create a comprehensive personality profile. This profile will then be checked against the job requirements to understand the best personality fit for the role and rank candidates accordingly. Through Appliqant’s platform, candidates are profiled on the Big 5 OCEAN characteristics – openness, conscientiousness, extraversion, agreeableness, and neuroticism.
Algorithms are able to detect facial expressions in recorded interviews, including when someone is smiling or frowning. Appliqant’s technology is always evolving, as we are currently working to measure these facial expressions against pre-set questions and phrases. This will allow you to see how a candidate reacts to each question and create a more robust understanding of their experience and working style.
What’s Next
The ultimate goal of integrating AI is to have a robot interviewer lead a natural-feeling interview for every candidate. The machine will be able to speak to a person and evaluate their skills and personality accurately every time.
Our AI technology is currently learning how to evaluate changes in a person’s expressions based on verbal and visual clues. This mimics the way humans form opinions about people, looking at their facial cues and body signals to understand their mood and disposition.
By utilizing AI to analyze automated video interviews, we are revolutionizing the recruitment industry to reduce waste in time and resources and bring you the best quality candidates for every position. Schedule your product demo today to learn how Appliqant can optimize your recruiting strategy.
—
Appliqant is an AI-infused, blockchain driven, automated video interview platform developed by the team at Quantilus.
Can Computers Create Art?
This paper discusses whether computers, using Artifical Intelligence (AI), could create art. The first part concerns AI-based tools for assisting with art making. The history of technologies that automated aspects of art is covered, including photography and animation. In each case, we see initial fears and denial of the technology, followed by acceptance, and a blossoming of new creative and professional opportunities for artists. The second part concerns AI systems that could conceive of artwork, and be credited with authorship of an artwork.